Let’s embark on an enthralling journey through the history of automatic music transcription (AMT), starting way back in 1897 with Karl Ferdinand Braun’s invention of the first analog oscilloscope. An oscilloscope is a fascinating device that makes audio visible by displaying sound waves as visual waveforms. This technology was pivotal in understanding the structure and dynamics of sound, paving the way for future advancements. It allowed us to see music, a crucial step towards translating audio into written notation.
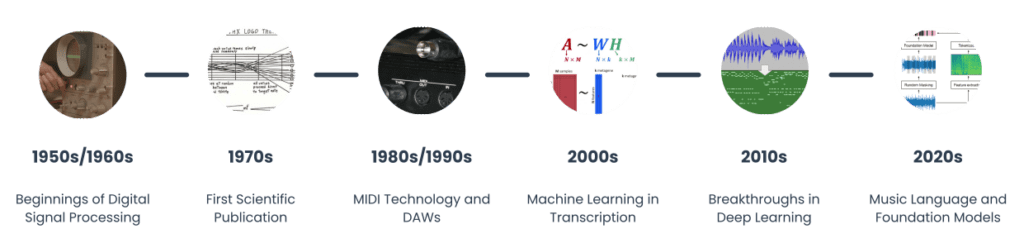
As we dive into the world of AMT, we’ll explore how it evolved from such early innovations to today’s sophisticated AI models. Ready to unravel the secrets of those notes on your screen and see how technology transformed them from mere sound waves to the music sheets we read today? Let’s jump in!
Beginnings of Digital Signal Processing
Rewind to the 1950s/1960s, an era bustling with technological innovation, where music took its first digital steps. Enter Digital Signal Processing (DSP), a revolutionary concept that employed techniques like Fast Fourier Transform (FFT). Imagine FFT as a musical mathematician, adeptly translating complex sound waves into decipherable data.
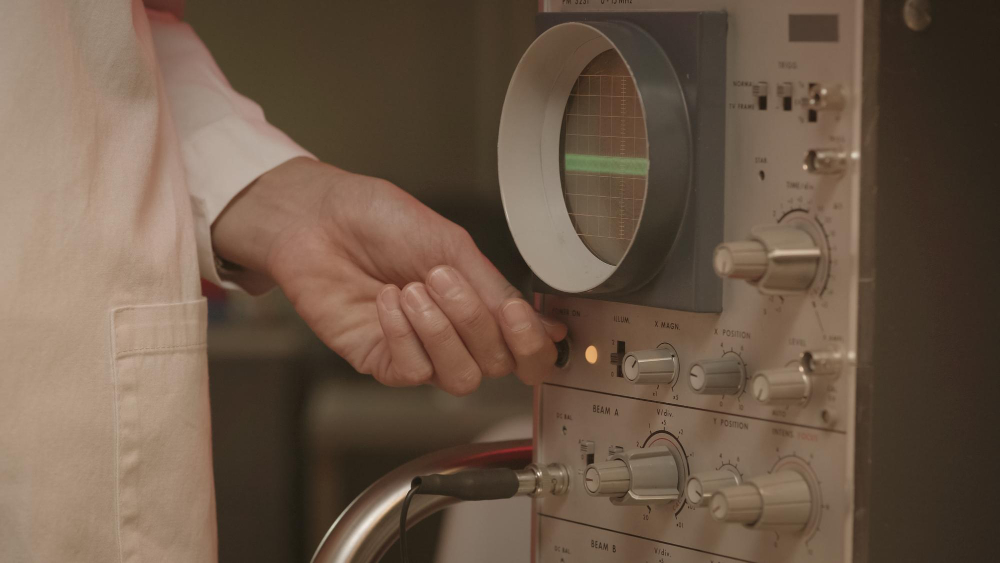
In 1977, James A. Moorer published the first scientific paper with the title “On the Transcription of Musical Sound by Computer” (link). In this paper, he introduces the proplem of automatic music transcription and provides a first rudimentary approach to tackle it. As a little fun fact: James A. Moorer also created the well known Deep Note audio logo of THX (the certification authority for cinemas founded by George Lucas).
MIDI and the Digital Revolution
Fast forward to the vibrant 1980s/1990s, a period marked by the dawn of MIDI (Musical Instrument Digital Interface) – a milestone in music technology. MIDI revolutionized how we interact with music, allowing diverse electronic instruments and computers to connect, communicate, and create.
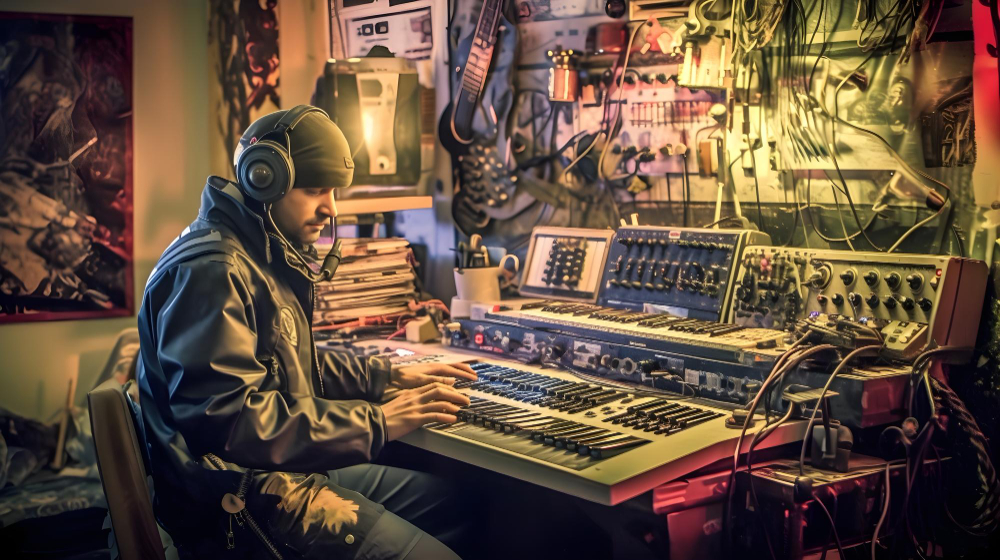
This era also saw the rise of digital audio workstations (DAWs), which made music production more accessible and versatile. For musicians, this was a golden era of creative liberation. Imagine being able to digitally orchestrate your compositions, capturing the essence of your musical thoughts with precision and ease. MIDI and DAWs democratized music production, breaking down barriers and allowing musicians from all backgrounds to experiment and produce music in ways previously unimaginable. This period was pivotal, not just in the evolution of music technology but in shaping the way we create, experience, and share music today.
The Age of Machine Learning
As we entered the 2000s, the AMT landscape buzzed with a new player: machine learning. This wasn’t just a step forward; it was a leap into a future where technology could learn and adapt. Machine learning approaches in AMT meant systems could now improve their understanding of music based on data, enhancing note recognition and transcription accuracy. Imagine having a smart assistant, not just programmed to follow rules, but capable of learning from every piece of music it encounters. This breakthrough was significant for musicians and music enthusiasts alike. It opened up possibilities for more accurate and nuanced transcriptions, enabling a deeper engagement with music. Whether you were deciphering a complex jazz improvisation or a classical sonata, machine learning was transforming the way we transcribed and interacted with music, making it a more intuitive and responsive process.
Rise of Deep Learning in AMT
The 2010s heralded the era of deep learning, taking the capabilities of machine learning to unprecedented heights. Deep learning models, with their intricate neural networks, began to dissect and understand complex musical patterns and nuances like never before. This advancement in AMT wasn’t just about accuracy; it was about depth and richness in transcription. For us musicians, it was akin to having a seasoned music transcriber by our side, one who could grasp the subtleties of rhythm, melody, and harmony in intricate detail. The implications were profound – not only did it streamline the transcription process, but it also opened up new avenues for musical analysis and creativity. Deep learning in AMT allowed us to delve deeper into the intricacies of music, understanding and interpreting compositions with a level of precision and insight that was previously unattainable.
The Current Era: Music Language and Foundation Models
In the 2020s, we are witnessing the advent of music language models and foundation models, the latest frontiers in AI music transcription. These models go beyond mere note transcription; they understand music’s emotional and stylistic nuances. Imagine a system that doesn’t just transcribe notes but captures the mood, the feel, and the essence of a piece. This era is about bridging the gap between human creativity and technological innovation. For musicians, this means tools that not only transcribe but also inspire and enhance creativity. These advanced AI models offer a more holistic understanding of music, making them invaluable allies in our creative journeys. They represent the pinnacle of how technology can complement and elevate the art of music, providing us with insights and capabilities that enrich our musical experiences and creations.
Conclusion
From the initial forays into DSP and the quaint oscilloscopes of the mid-20th century to today’s advanced AI models, the journey of AMT is nothing short of extraordinary. It’s a narrative of constant innovation and evolution, where each chapter adds depth and dimension to our understanding and interaction with music. As musicians, embracing these technologies doesn’t just mean adopting new tools; it’s about